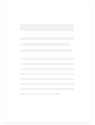
自引率: 18.9%
被引量: 4178
通过率: 暂无数据
审稿周期: 6
版面费用: 暂无数据
国人发稿量: 17
投稿须知/期刊简介:
Water Environment Research (WER) is a peer-reviewed environmental journal for the dissemination of fundamental and applied research in all scientific and technical areas related to water quality and pollution control. Topics of interest include hazardous wastes, ground and surface waters, drinking water, source water protection, remediation and treatment systems, conservation and reuse, environmental risk and health, biosolids and residuals management, waste minimization, and aquatic sciences. Water Environment Research seeks to discuss complete and fully interpreted results of original research and to report studies of engineering and science applicability.
-
Understanding machine learning predictions of wastewater treatment plant sludge with explainable artificial intelligence.
This study investigates the use of machine learning (ML) models for wastewater treatment plant (WWTP) sludge predictions and explainable artificial intelligence (XAI) techniques for understanding the impact of variables behind the prediction. Three ML models, random forest (RF), gradient boosting machine (GBM), and gradient boosting tree (GBT), were evaluated for their performance using statistical indicators. Input variable combinations were selected through different feature selection (FS) methods. XAI techniques were employed to enhance the interpretability and transparency of ML models. The results suggest that prediction accuracy depends on the choice of model and the number of variables. XAI techniques were found to be effective in interpreting the decisions made by each ML model. This study provides an example of using ML models in sludge production prediction and interpreting models applying XAI to understand the factors influencing it. Understandable interpretation of ML model prediction can facilitate targeted interventions for process optimization and improve the efficiency and sustainability of wastewater treatment processes. PRACTITIONER POINTS: Explainable artificial intelligence can play a crucial role in promoting trust between machine learning models and their real-world applications. Widely practiced machine learning models were used to predict sludge production of a United States wastewater treatment plant. Feature selection methods can reduce the required number of input variables without compromising model accuracy. Explainable artificial intelligence techniques can explain driving variables behind machine learning prediction.
被引量:- 发表:2024
-
Characterization and spatial distribution of microplastics in Surma river, Bangladesh: Assessing water and sediment dynamics.
被引量:- 发表:2024
-
Gravity-driven packed bed filter, with copper-impregnated activated carbon, for continuous water disinfection in absence of electricity.
被引量:- 发表:2024
-
Social dimensions of climate-induced flooding in Jakarta (Indonesia): The role of non-point source pollution.
被引量:- 发表:2024
-
Analysis and calculation of scour critical velocity of suspended particles in a storm sewer.
被引量:- 发表:2024