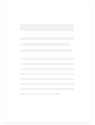
自引率: 3.6%
被引量: 4973
通过率: 暂无数据
审稿周期: 暂无数据
版面费用: 暂无数据
国人发稿量: 34
投稿须知/期刊简介:
The Journal of Computer Assisted Tomography (JCAT) is the only major periodical devoted exclusively to tomographic reconstructive imaging. It publishes original articles, reports, and studies that describe the latest clinical and research developments in computer-based diagnostic imaging. Each issue of this journal contains as many as 20 original articles on a wide range of pathologic conditions.
期刊描述简介:
The Journal of Computer Assisted Tomography (JCAT) is the only major periodical devoted exclusively to tomographic reconstructive imaging. It publishes original articles, reports, and studies that describe the latest clinical and research developments in computer-based diagnostic imaging. Each issue of this journal contains as many as 20 original articles on a wide range of pathologic conditions.
-
Coronary Computed Tomography Angiography Using an Optimal Acquisition Time Window Based on Heart Rate Determined During Breath-Holding Following Free Breathing.
被引量:- 发表:1970
-
Changes of the Buccal Fat Pad Volume According to the Different Age Groups, Gender, and Body Mass Index: An Evaluation With Computed Tomography.
被引量:- 发表:1970
-
Evaluation of Pericoronary Fat Attenuation Index to Better Identify Culprit Lesions in Acute Coronary Syndrome According to Stenosis Severity.
被引量:- 发表:1970
-
Reverse Encoding Distortion Correction for Clinical Head Echo-Planar Diffusion-Weighted MRI: Initial Experience.
This study aimed to evaluate the feasibility of the recently commercialized reverse encoding distortion correction (RDC) method for echo-planar imaging (EPI) diffusion-weighted imaging (DWI) by applying clinical head MRI. This study included 50 consecutive patients who underwent head MRI, including single-shot (SS) EPI DWI and RDC-EPI DWI. For evaluation of normal structures, qualitative scores for image distortion, Dice similarity coefficient (DSC) values, distortion ratios, and mean apparent diffusion coefficient (ADC) values were assessed in the pons, temporal lobe at the skull base, and frontal lobe at the level of the lateral ventricles in 30 patients. To evaluate pathologies, qualitative scores for image distortion were assessed for 25 intracranial and 21 extracranial pathologies identified in 32 patients. Qualitative scores for image distortion, DSC values, distortion ratios, and mean ADC values of the pons and temporal lobe were significantly different between SS-EPI DWI and RDC-EPI DWI, whereas those of the frontal lobe at the level of the lateral ventricles were not significantly different between the 2 DWIs. The qualitative scores for image distortion and mean ADC values of extracranial pathologies were significantly different between the DWIs, whereas those of intracranial pathologies were not significantly different. RDC-EPI DWI significantly reduced image distortion and showed higher mean ADC values of the brain parenchyma in the skull base and extracranial pathologies.
被引量:- 发表:1970
-
Application of U-Net Network Utilizing Multiattention Gate for MRI Segmentation of Brain Tumors.
Studies have shown that the type of low-grade glioma is associated with its shape. The traditional diagnostic method involves extraction of the tumor shape from MRIs and diagnosing the type of glioma based on corresponding relationship between the glioma shape and type. This method is affected by the MRI background, tumor pixel size, and doctors' professional level, leading to misdiagnoses and missed diagnoses. With the help of deep learning algorithms, the shape of a glioma can be automatically segmented, thereby assisting doctors to focus more on the diagnosis of glioma and improving diagnostic efficiency. The segmentation of glioma MRIs using traditional deep learning algorithms exhibits limited accuracy, thereby impeding the effectiveness of assisting doctors in the diagnosis. The primary objective of our research is to facilitate the segmentation of low-grade glioma MRIs for medical practitioners through the utilization of deep learning algorithms. In this study, a UNet glioma segmentation network that incorporates multiattention gates was proposed to address this limitation. The UNet-based algorithm in the coding part integrated the attention gate into the hierarchical structure of the network to suppress the features of irrelevant regions and reduce the feature redundancy. In the decoding part, by adding attention gates in the fusion process of low- and high-level features, important feature information was highlighted, model parameters were reduced, and model sensitivity and accuracy were improved. The network model performed image segmentation on the glioma MRI dataset, and the accuracy and average intersection ratio (mIoU) of the algorithm segmentation reached 99.7%, 87.3%, 99.7%, and 87.6%. Compared with the UNet, PSPNet, and Attention UNet network models, this network model has obvious advantages in accuracy, mIoU, and loss convergence. It can serve as a standard for assisting doctors in diagnosis.
被引量:- 发表:1970